In July, 2023, Science Gallery London and the London Office of Technology and Innovation co-hosted a workshop helping Londoners think about the kind of AI they want. In this post, Dr. Peter Rees reflects on the event, describes its methodology, and celebrates some of the new images that resulted from the day.
Who can create better images of Artificial Intelligence (AI)? There are common misleading tropes of the images which dominate our culture such as white humanoid robots, glowing blue brains, and various iterations of the extinction of humanity. Better Images of AI is on a mission to increase AI literacy and inclusion by countering unhelpful images. Everyone should get a say in what AI looks like and how they want to make it work for them. No one perspective or group should dominate how Al is conceptualised and imagined.
This is why we were delighted to be able to run the workshop ‘Co-creating Better Images of AI’ during London Data Week. It was a chance to bring together over 50 members of the public, including creative artists, technologists, and local government representatives to each make our own images of AI. Most images of AI that appear online and in the newspapers are copied directly from existing stock image libraries. This workshop set out to see what would happen when we created new images fromscratch. We experimented with creative drawing techniques and collaborative dialogues to create images. Participants’ amazing imaginations and expertise went into a melting-pot which produced an array of outputs. This blogpost reports on a selection of the visual and conceptual takeaways! I offer this account as a personal recollection of the workshop—I can only hope to capture some of the main themes and moments, and I apologise for all that I have left out.
The event was held at the Science Gallery in London on 4th July 2023 between 3-5pm and was hosted in partnership with London Data Week, funded by the London Office of Innovation and Technology. In keeping with the focus on London Data Week and LOTI, the workshop set out to think about how AI is used every day in the lives of Londoners, to help Londoners think about the kind of AI they want, to re-imagine AI so that we can build systems that work for us.
Workshop methodology
I said the workshop started out from scratch—well, almost. We certainly wanted to make use of the resources already out there such as the [Better Images of AI: A Guide for Users and Creators] co-authored by Dr Kanta Dihal and Tania Duarte. This guide was helpful because it not only suggested some things to avoid, but also provided stimulation for what kind of images we might like to make instead. What made the workshop a success was the wide-ranging and generous contributions—verbal and visual—from invited artists and technology experts, as well as public participants, who all offered insights and produced images, some of which can be found below (or even in the Science Gallery).
The Workshop was structured in two rounds, each with a live discussion and creative drawing ‘challenge’. The approach was to stage a discussion between an artist and a technology expert (approx 15 mins), and then all members of the workshop would have some time (again, approx 15 mins) for creative drawing. The purpose of the live discussion was to provide an accessible introduction to the topic and its challenges, after which we all tackled the challenge of visualising and representing different elements of AI production, use and impact. I will now briefly describe these dialogues, and unveil some of the images created.
Setting the scene
Tania Duarte (Founder, We and AI) launched the workshop with a warm welcome to all. Then, workshop host Dr Robert Elliot-Smith (Director of AI and Data Science at Digital Catapult) introduced the topic of Large Language Models (LLMs) by reminding the audience that such systems are like ‘autocorrect on steroids’: the model is simply very good at predicting words, it does not have any deep understanding of the meaning of the text it produces. He also discussed image-generators, which work in a similar way and with similar problems, which is why certain AI-produced images end up garbling images of hands and arms: they do not understand anatomy.
In response to this preliminary introduction, one participant who described herself as a visual artist expressed horror at the power of such image-generating and labelling AI systems to limit and constrain our perception of reality itself. She described how, if we are to behave as artists, what we have to do in our minds is to avoid seeing everything simply in terms of fixed categories which can conservatively restrain the imagination, keeping it within a set of known categorisations, which is limiting not only our imagination but also our future. For instance, why is the thing we see in front of us necessarily a ‘wall’? Could it not be, seeing more abstractly, simply a straight line?
From her perspective, AI models seem to be frighteningly powerful mechanisms for reinforcing existing categories for what we are seeing, and therefore also of how to see, what things are, even what we are, and what kind of behaviour is expected. Another participant agreed: it is frustrating to get the same picture from 100 different inputs and they all look so similar. Indeed, image generators might seem to be producing novelty, but there is an important sense in which they are reinforcing the past categories of the data on which they were trained.
This discussion raised big questions leading into the first challenge: the limitations of large language models.
Round 1: The Limitations of Large Language Models.
A live discussion was staged between Yasmine Boudiaf (recognised as one of ‘100 Brilliant Women in AI Ethics 2022,’ and fellow at the Ada Lovelace Institute) and Tamsin Nooney (AI Research, BBC R&D) about the process of creating LLMs.
Yasmine asked Tamsin about how the BBC, as a public broadcaster, can use LLMs in a reliable manner, and invited everyone in the room to note down any words they found intriguing, as those words might form a stimulus for their creative drawings.
Tamsin described an example of LLM use-case for the BBC in producing a podcast whereby an LLM could summarise the content, add in key markers and meta-data labels and help to process the content. She emphasised how rigorous testing is required to gain confidence in the LLM’s reliability for a specific task before it could be used. A risk is that a lot of work might go into developing the model only for it to never be usable at all.
Following Yasmine’s line of question, Tamsin described how the BBC deal with the significant costs and environmental impacts of using LLMs. She described how the BBC calculated if they wanted to train their LLM, even a very small one, it would take up all their servers at full capacity for over a year, so they won’t do that! The alternative is then to pay other services such as Amazon to use their model, which means balancing costs: so here are limits due to scale, cost, and environmental impact.
This was followed by a more quiet, but by no means silent, 15 minutes for drawing time in which all participants drew…
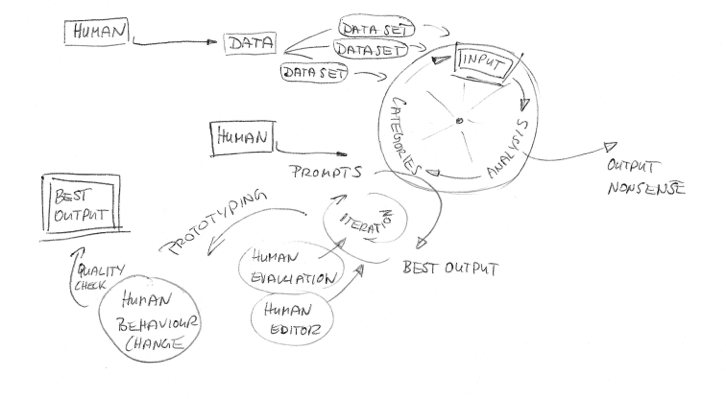
One participant used an AI image generator for their creative drawing, making a picture of a toddler covered in paint to depict the LLM and its unpredictable behaviours. Tamsin suggested that this might be giving the LLM too much credit! Toddlers, like cats and dogs, have a basic and embodied perception of the world and base knowledge, which LLMs do not have.
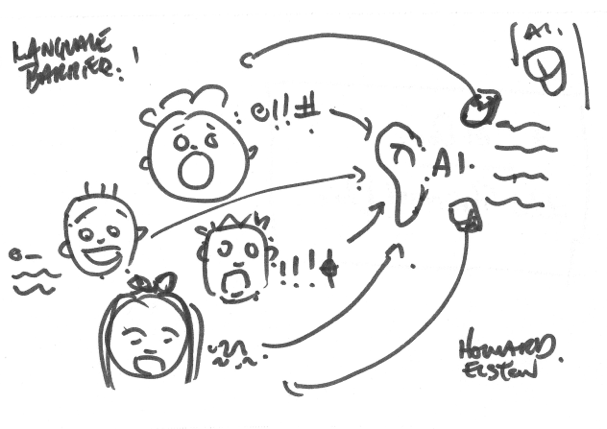
The experience of this discussion and drawing also raised, for another participant, more big questions. She discussed poet David Whyte’s work on the ‘conversational nature of reality’ and thought on how the self is not just inside us but is created through interaction with others and through language. For instance, she mentioned that when you read or hear the word ‘yes’, you have a physical feeling of ‘yesness’ inside, and similarly for ‘no’. She suggested that our encounters with machine-made language produced by LLMs is similar. This language shapes our conversations and interactions, so there is a sense in which the ‘transformers’ (the technical term for the LLM machinery) is also helping to transform our senses of self and the boundary between what is reality and what is fantasy.
Here, we have the image made by artist Yasmine based on her discussion with Tamsin:
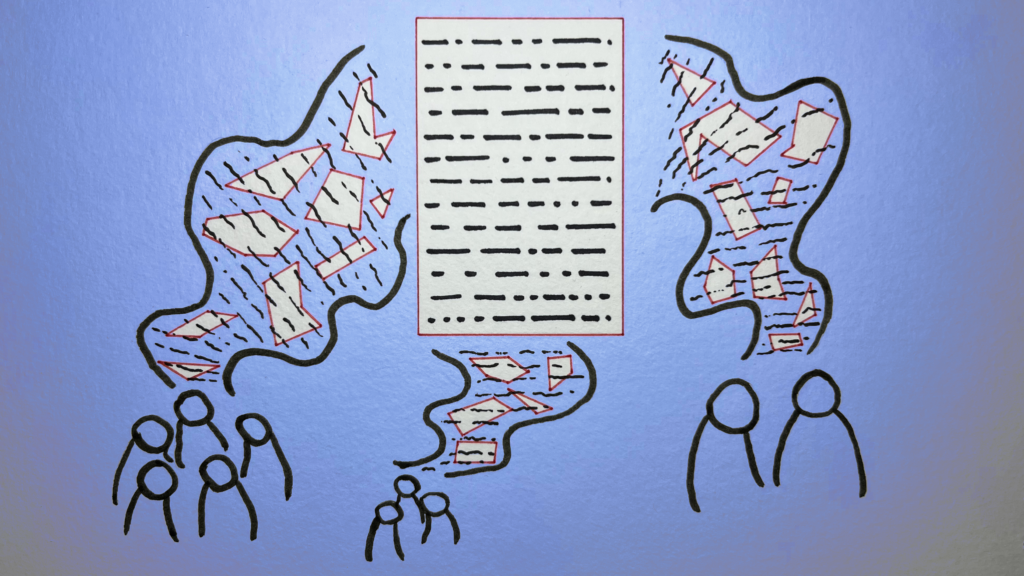
Yasmine writes:
This image shows an example of Large Language Model in use. Audio data is gathered from a group of people in a meeting. Their speech is automatically transcribed into text data. The text is analysed and relevant segments are selected. The output generated is a short summary text of the meeting. It was inspired by BBC R&D’s process for segmenting podcasts, GPT-4 text summary tools and LOTI’s vision for taking minutes at meetings.
Yasmine Boudiaf
You can now find this image in the Better Images of AI library, and use it with the appropriate attribution: Image by Yasmine Boudiaf / © LOTI / Better Images of AI / Data Processing / CC-BY 4.0. With the first challenge complete, it was time for the second round.
Round 2: Generative AI in Public Services
This second and final round focused on use cases for generative AI in the public sector, specifically by local government. Again, a live discussion was held, this time between Emily Rand (illustrator and author of seven books and recognised by the Children’s Laureate, Lauren Child, to be featured in Drawing Words) and Sam Nutt (Researcher & Data Ethicist, London Office of Technology and Innovation). They built on the previous exploration of LLMs by considering new generative AI applications which they enable for local councils and how they might transform our everyday services.
Emily described how she illustrates by hand, and described her [work] as focusing on the tangible and the real. Making illustrations about AI, whose workings are not obviously visible, was an exciting new topic. See her illustration and commentary below.
Sam described his role as part of the innovation team which sits across 26 of the boroughs of London and Mayor of London. He helps boroughs to think about how to use data responsibly. In the context of local government data and services, a lot of data collected about residents is statutory (meaning they cannot opt out of giving it), such as council tax data. There is a big prerogative for dealing with such data, especially for sensitive personal health data, that privacy is protected and bias is minimised. He considered some use cases. For instance, council officers can use ChatGPT to draft letters to residents to increase efficiency butthey must not put any personal information into ChatGPT, otherwise data privacy can be compromised. Or, for example, the use of LLMs to summarise large archives of local government data concerning planning permission applications, or the minutes from council meetings, which are lengthy and often technical, which could be made significantly more accessible to many members of the public and researchers.
Sam also raised the concern that it is very important that residents know how councils use their data so that councils can be held accountable. Therefore this has to be explained and made understandable to residents. Note that 3% of Londoners are totally offline, not using internet at all, so that’s 270,000 people—who also have an equal right to understand how the council uses their data—who need to be reached through offline means. This example brings home the importance of increasing inclusive public Al literacy.
Again, we all drew. Here are a couple of striking images made by participants who also kindly donated their pictures and words to the project:

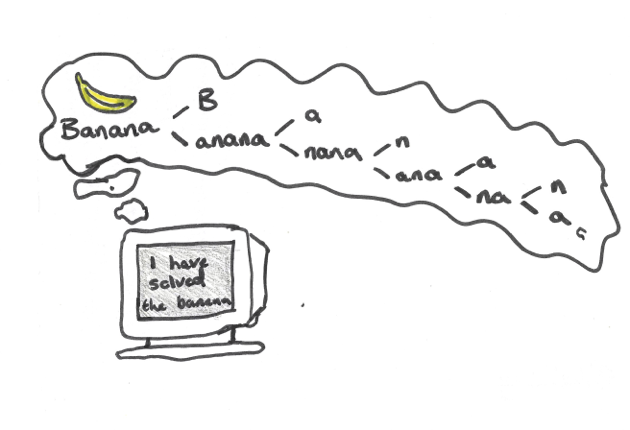
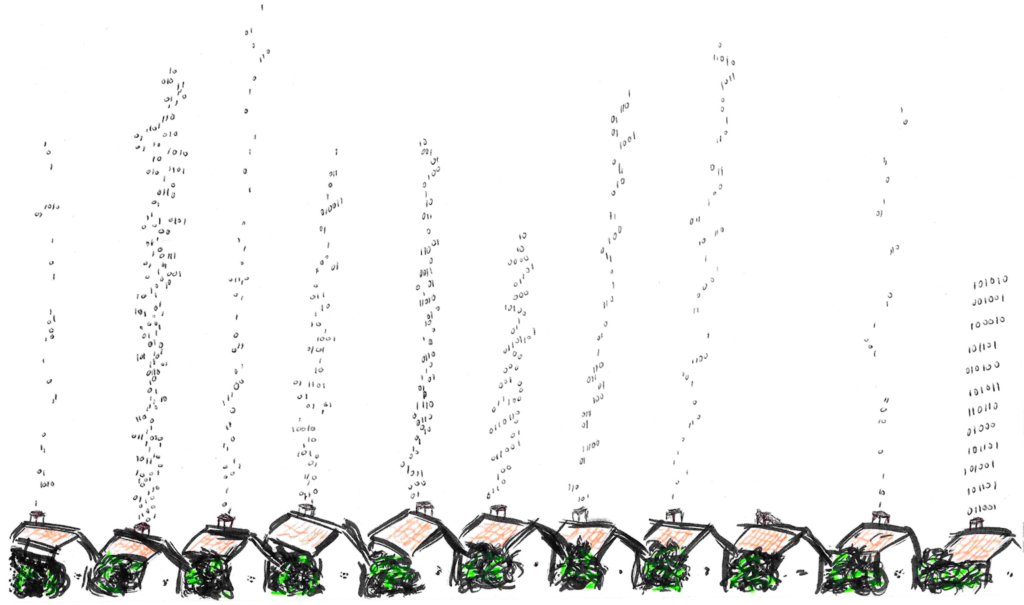
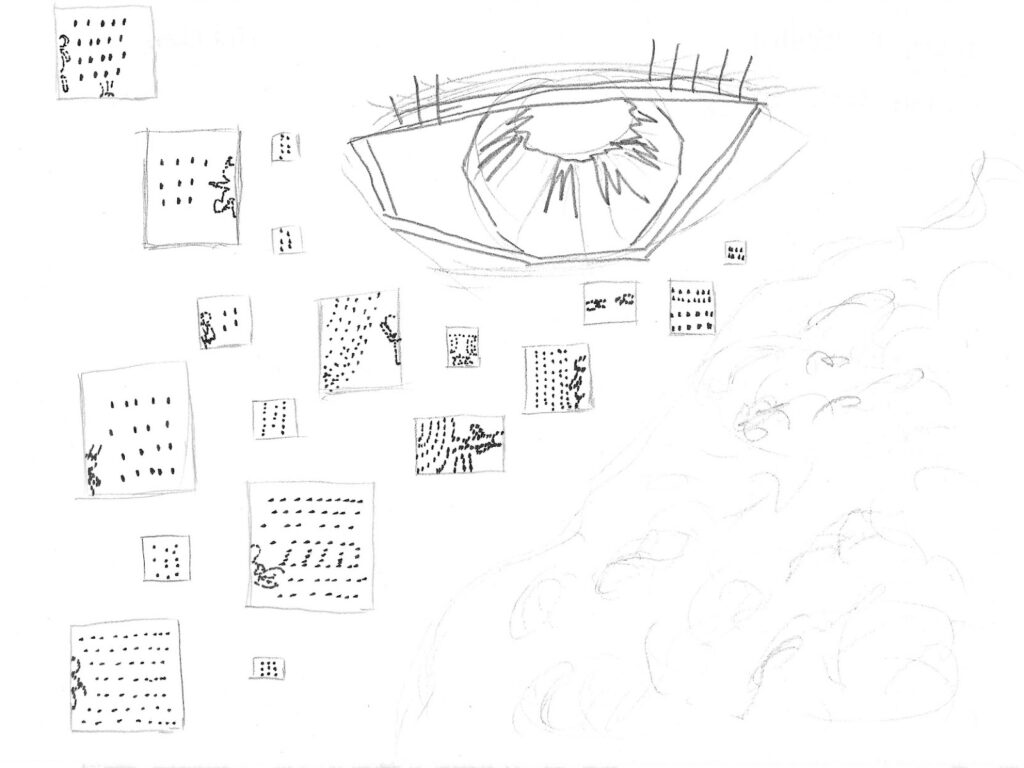

Emily started by llustrating the idea of bias in AI. Her initial sketches showed an image showing lines of people of various sizes, ages, ethnicities and bodies. Various cursors showed the cis white able bodied people being selected over the others. Emily also did a sketch of the shape of a City and ended up combining the two. She added frames to show the way different people are clustered. The frame shows the area around the person, where they might have a device sending data about them.
Emily’s final illustration is below, and can be downloaded from here and used for free with the correct attribution Image by Emily Rand / © LOTI / Better Images of AI / AI City / CC-BY 4.0.

At the end of the workshop, I was left with feelings of admiration and positivity. Admiration of the stunning array of visual and conceptual responses from participants, and in particular the candid and open manner of their sharing. And positivity because the responses were often highlighting the dangers of AI as well as the benefits—its capacity to reinforce systemic bias and aid exploitation—but these critiques did not tend to be delivered in an elegiac or sad tone, they seemed more like an optimistic desire to understand the technology and make it work in an inclusive way. This seemed a powerful approach.
The results
The Better Images of AI mission is to create a free repository of better images of AI with more realistic, accurate, inclusive and diverse ways to represent AI. Was this workshop a success and how might it inform Better Images of AI work going forward?
Tania Duarte, who coordinates the Better Images of AI collaboration, certainly thought so:
It was great to see such a diverse group of people come together to find new and incredibly insightful and creative ways of explaining and visualising generative AI and its uses in the public sector. The process of questioning and exploring together showed the multitude of lenses and perspectives through which often misunderstood technologies can be considered. It resulted in a wealth of materials which the participants generously left with the project, and we aim to get some of these developed further to work on the metaphors and visual language further. We are very grateful for the time participants put in, and the ideas and drawings they donated to the project. The Better Images of AI project, as an unfunded non-profit is hugely reliant on volunteers and donated art, and it is a shame such work is so undervalued. Often stock image creators get paid $5 – $25 per image by the big image libraries, which is why they don’t have time to spend researching AI and considering these nuances, and instead copy existing stereotypical images.
Tania Duarte
The images created by Emily Rand and Yasmine Boudiaf are being added to the Better Images of AI Free images library on a Creative Commons licence as part of the #NewImageNovember campaign. We hope you will enjoy discovering a new creative interpretation each day of November, and will be able to use and share them as we double the size of the library in one month.
Sign up for our newsletter to get notified of new images here.
Acknowledgements
A big thank you to organisers, panellists and artists:
- Jennifer Ding – Senior Researcher for Research Applications at The Alan Turing Institute
- Yasmine Boudiaf – Fellow at Ada Lovelace Institute, recognised as one of ‘100 Brilliant Women in AI Ethics 2022’
- Dr Tamsin Nooney – AI Research, BBC R&D
- Emily Rand – illustrator and author of seven books and recognised by the Children’s Laureate, Lauren Child, to be featured in Drawing Words
- Sam Nutt – Researcher & Data Ethicist, London Office of Technology and Innovation (LOTI)
- Dr Tomasz Hollanek – Research Fellow, Leverhulme Centre for the Future of Intelligence
- Laura Purseglove – Producer and Curator at Science Gallery London
- Dr Robert Elliot-Smith – Director of AI and Data Science at Digital Catapult
- Tania Duarte – Founder, We and AI and Better Images of AI
Also many thanks to the We and Al team, who volunteered as facilitators to make this workshop possible:
- Medina Bakayeva, UCL master’s student in cyber policy & AI governance, communications background
- Marissa Ellis, Founder of Diversily.com, Inclusion Strategist & Speaker @diversily
- Valena Reich, MPhil in Ethics of AI, Gates Cambridge scholar-elect, researcher at We and AI
- Ismael Kherroubi Garcia FRSA, Founder and CEO of Kairoi, AI Ethics & Research Governance
- Dr Peter Rees was project manager for the workshop
And a final appreciation for our partners: LOTI, the Science Gallery London, and London Data Week, who made this possible.
Related article from BIoAI blog: ‘What do you think AI looks like?’: https://blog.betterimagesofai.org/what-do-children-think-ai-looks-like/